Making better use of existing video networks
Overcoming the challenge of manually analysing video feeds from security cameras is one of the biggest tasks for companies that use video surveillance on their premises.
Security agents have to spend many hours checking multiple computer screens, and oftentimes there are not sufficient agents to review all the screens. Even when there is enough human personnel, it is highly improbable that they will be able to identify every event.
What is a proper solution to ensure that every video feed is checked, at any time of the day, without missing on key events?
Recent advances in Artificial Intelligence and Deep Learning now allow for a streamlined and efficient manner of dealing with such large amounts of information coming from video feeds.
AI Video Analytics is an accurate technology that allows for real-time monitoring and detection of events, by transforming raw video data into intelligible video information.
Real-time monitoring and reporting
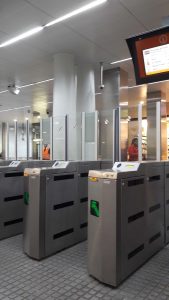
One example where the application of AI Video Analytics is proving effective is public transport.
With hundreds and even thousands of security cameras installed in some transit systems, it makes a difference security staff to have real-time situational awareness.
Fare evasion is a global issue that causes losses in billions of dollars for transport operators worldwide. In 2019, fare infraction losses amounted to € 500 million in France, $ 250 million in New York’s MTA, £100 million on London’s TfL, and A$ +80 million on Australia’s New South Wales.
AI Video Analytics can help to substantially reduce fare evasion at transport access nodes that use turnstiles or swing gates.
With the help of video cameras installed above the gates, an AI system can monitor and analyze the flow and passing of passengers. The system can trigger alerts when detecting anomalies such as fare infractions.
This type of system can function 24/7 with a constant level of efficiency. At the same time, after the training of the initial model is complete and reaches an acceptable degree of efficiency, it continues to upgrade and learn over time.
Such a system can be configured to send instant alerts to a mobile application on the smartphones of key personnel, allowing them to catch the offenders on the spot.
Optimising performance through data analysis
An AI Video Analytics system can aggregate data over time and statistically display it on dashboards or heatmaps.
Transport managers can use these insights to understand their operational performance and make informed, data-driven decisions.
For instance, if they find that certain sites have high fare evasion rates, they might decide to undertake extra research to know the reasons behind the fare infractions. Or they might decide to adjust fares or upgrade the technology behind the ticketing system. Alternatively, they can employ a public information campaign to raise awareness and inform the public about the issue.
The data from an AI system can also help with planning for future improvements or making decisions about the geometry of a station, improving passenger flow, or deploying new technology with a more preventive approach against fare evasion.
Smooth customer experience and ensuring the feeling of safety in public transport are key aspects that transport companies plan in their core annual strategies.
An AI system can help to create a powerful deterrent effect against fare evasion. This allows passengers to feel safer and enjoy a pleasant ride on public transit.